Previously on bedside cyborgs... Part 3
Welcome the grand finale of this three-part blog series on intelligent search in a healthcare context.
We kicked off this series by pointing to modern search technology as one of the defining innovations of the Information Age. In an era when we are creating mind-boggling quantities of data every day, search engines help us make sense of this digital jungle, placing a world of knowledge at our fingertips. Many of us have come to depend on them in both our work and personal lives, leading Elon Musk to famously claim that we have become a society of cyborgs.
In the first post of the series, we outlined the impact that bleeding edge search technology could have on modern healthcare practice… if only we could link it up with our non-internet-based data. In the second post, we explored some of the modern AI technology that has fuelled big leaps in search capabilities over recent years. We also noted that this technology requires very specialised infrastructure, which doesn’t mix well with the traditional paradigm of “on-prem” computing in healthcare. We wrapped up with a quick look at cloud computing as an alternative framework for health information management.
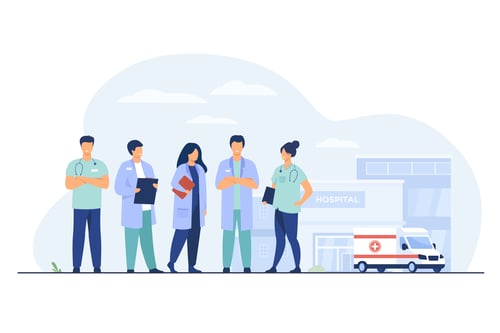
In this post, we’ll be picking up on that latter topic. But before we go any further, it’s worth noting that the scope of this problem is much broader than we’ve suggested so far.
Search is a particularly impactful use-case for AI in healthcare, which is why we’ve dedicated a blog series to it. But any applications that use AI under the hood are likely to face similar challenges in the clinical domain. This includes AI for image processing in specialties like radiology, pathology, dermatology, ophthalmology, etc., which is already starting to become more mainstream. It also includes more nascent applications like AI for real-time patient monitoring, length of stay prediction, readmission risk assessment, and so on.
With AI in healthcare set to become a $45BN market over the next few years, health strategists pointing to digital innovation as a pillar of 21st century healthcare, and the world’s largest clinical organisations like the NHS busy developing AI strategies, this isn’t a problem healthcare executives can ignore and hope it will go away. So in this post, we’ll open up the discussion a little to encompass AI in healthcare as a whole, rather than just AI-enabled search, before we return our focus to the latter for the wrap-up.
Without further ado, then, let’s dive back into the world of cloud versus on-prem computing.
Why wouldn’t you migrate health data to the cloud?
In the last post, we covered some of the key benefits of cloud computing, including the fact that it's much easier to access AI-ready infrastructure in the cloud than provision it locally. But there are significant downsides too.
Firstly, if you’re dealing with highly sensitive material like personal health information (often referred to as PHI for short), entrusting it to a third party should be done with caution. Once upon a time, the main concern about moving sensitive data might have been cybersecurity. But modern encryption methods are extremely robust, and that’s likely to remain the case until quantum computers become advanced enough to break current encryption methods - probably many years away. And at that point, we may well have access to new encryption methods based on quantum entanglement, which is something the Chinese government has already pioneered by sending quantum encrypted messages into space and back.
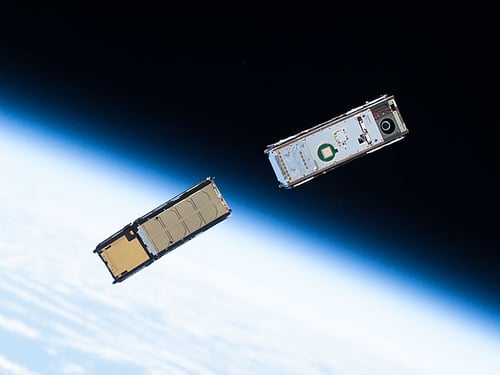
Rather, the issue with migrating PHI to the public cloud is trust. From a European healthcare provider’s perspective, it doesn’t help that the three players that currently dominate the public cloud market are US tech giants Microsoft, Google and Amazon. This can play poorly with patients, especially given that at least one of these companies has been the subject of bad press involving NHS data in the past. But it can also create legal problems: the fact that US law enforcement agencies can gain access to data housed by US companies (under the CLOUD Act) recently led the German government to effectively ban US cloud providers from hosting German healthcare data.
Secondly, the cloud isn’t always cheaper. Accessing your own organisation’s data on the cloud means sending it back and forth across the internet, which incurs charges. If you have large files (for example, digital pathology images) that you need to access regularly, it can be more cost-effective to keep them within your own local network. It can also be expensive to migrate between cloud service providers, so if your provider does something you don’t like - ups their prices, for example, or changes the way they handle sensitive data - you could find that it’s difficult to vote with your feet.
Lastly, and probably most importantly, migrating to the cloud can be disruptive. Most healthcare systems worldwide are scrambling to get on top of the care backlog created by the pandemic. In the case of the NHS, this is compounded by a ‘staggering’ vacancy rate of almost 10%, leading UK Parliament’s own health and social care committee to warn that persistent understaffing in the NHS poses a serious risk to staff and patient safety. Even if migrating to the cloud is key to enabling innovative new technology, the short-term pain of major digital transformation may seem unconscionable right now, and the healthcare sector's digital skills gap doesn't make things any easier.
Fortunately, ‘migrating to the cloud’ doesn’t have to be an all-or-nothing thing. If you’re planning to move your entire electronic health record to a cloud service provider then sure, it’s probably going to be a big bang affair. But there are plenty of other areas where data and applications can be outsourced in bite size chunks, as and when it makes sense. As an example, I’ll briefly outline our approach here at Eolas Medical.
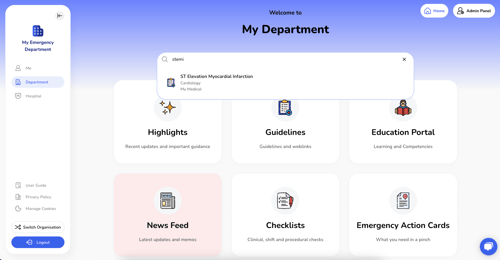
How is Eolas Medical using the cloud to connect healthcare professionals with the information they need?
Eolas is a cloud-based content management service for healthcare providers. Our bread and butter is local guidelines, clinical pathways, policy documents, training and education materials for frontline staff, rotas, etc. In other words, all the non-PHI that a healthcare provider needs to place at their staff’s fingertips at the point of care.
This kind of content is a great place to start building a cloud footprint, for several reasons. Firstly, there are lots of ways to break it up into bitesize chunks and migrate gradually. Secondly, the data is often commercially sensitive, but still a lot easier to move off site than PHI. Thirdly, the benefits are immediate.
For example, once content is uploaded to our servers we can deliver it to any device our users want. This sounds almost trivial, but it can make a big difference. Once a hospital's content is migrated to our platform, it is accessed by smartphone over 90% of the time, so it's clear that frontline healthcare staff strongly prefer this. Being cloud-based also means that we can easily push updates to all our users at once, whether they work at Stanford or St George’s. This allows us to respond rapidly to feedback and continually refine the platform for our users' needs - a key reason that clinicians tell us they find our apps so intuitive.
Most importantly for today's discussion, the cloud allows us to deploy modern search technology without creating a headache for hospital IT teams. This is the secret source that allows us to connect clinical users with medical information super-fast, and is a big part of why Dr Neill Hughes from Salford Royal’s Emergency Department described our platform “a game changer for productivity”.
All of that said, we're very aware that what we do is only one part of a much bigger jigsaw. The reason we stick to non-patient data is so that we can make a difference here and now. As we noted in part one of this series, applying advanced information management strategies like ours to PHI could create some huge benefits. But there are also significant barriers to doing that. Let's take a look at some of those now.
What are the key barriers to using artificial intelligence with personal health information?
1. Infrastructure.
Earlier this year, the Goldacre review noted that most UK patient data is still stored in NHS-owned data centres with limited capability for advanced analytics, which includes AI. Moreover, the size and computational intensity of state-of-the-art AI algorithms has grown exponentially over the last couple of years, so that gulf is only widening.
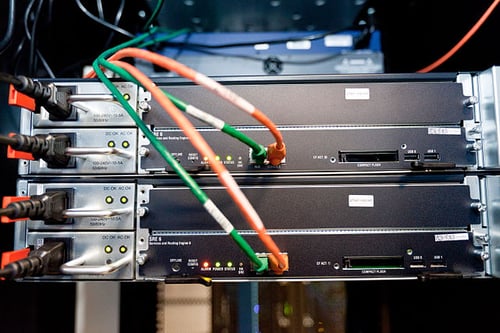
2. Data governance.
This comes in two parts: the governance of putting PHI into the cloud, and the governance of exposing it to AI. Although the former is slowly being addressed by consensus recommendations like those in the NHS and social care data: off-shoring and the use of public cloud services guidance, it’s not a solved problem. The responsibility for data safeguarding still lies with local healthcare providers, where stories like the German health data embargo on US cloud providers might encourage administrators to sit tight until cloud migration of PHI is a better-trodden path.
In terms of exposing PHI to AI: we’ve already seen during this series that modern AI algorithms “read” web pages and other data sources. They can also retain enough of what they see during training to produce meaningful discourse on complex subjects, like GPT-3's article in the Guardian. So what happens when an AI “reads” through patient notes? For a response to that question,, you can refer to this 150 page report on the Ethics and Governance of AI for Health from the World Health Organisation. But in nutshell: it's all a bit complicated.
3. Domain shift.
Imagine what would happen if you asked someone with a PhD in astrophysics to step in for the triage nurse in the emergency department. Well, that’s loosely analogous to what might happen if you asked an AI trained on the “general internet” to make sense of clinical notes. We call this domain shift.
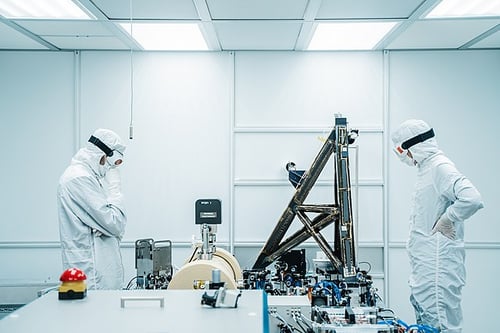
There are two reasons that domain shift is a particular problem in healthcare. Firstly, AI-based NLP algorithms have limited vocabularies. At the time of writing, many algorithms are capped at 50,000 words. (Technically, it’s actually 50,000 “tokens” - a term we covered in the last blog. But we’ll not worry about that for now.) Usually, the 50,000 words that appear most often in the training data are assigned to those vocabulary slots. The vocabulary is then fixed for the life of that algorithm. If your training data comes from, say, Wikipedia, Twitter, and other cross-domain sources, medical terms are unlikely to make the cut. This permanently limits the algorithm’s ability to make sense of medical language.
Secondly, words in natural language can be highly polysemous, which means they can have hundreds of meanings that vary with context, and polysemy is a particular challenge in the medical domain. An algorithm’s capacity for storing semantic information is limited - determined largely by the number of neural connections it contains and the size (or “dimensionality”) of its word embeddings. Consequently, when faced with a polysemous word, an AI will tend to prioritise the meanings that it encounters most frequently. Therefore, if you want your AI to perform well in a particular field or industry, you have to make sure you train it using plenty of data from that domain.
All in all, this means that AI algorithms trained using cross-domain data tend to perform poorly in a clinical setting. In fact, even algorithms trained on biomedical data such as PubMed articles struggle when it comes to clinical notes. This creates something of a chicken-and-egg situation. If we want to motivate administrators and policymakers to address the barriers to using AI with PHI, we need great point-of-care applications to make a strong business case. But to develop great applications, we need to train AI with PHI… And so on.
But don’t worry, we’re not going to end on a low note. Plenty of people have been giving these challenges a lot of thought. Below are a few of the solutions that have been mooted:
How can we overcome barriers to using artificial intelligence with personal health information?
1. Embrace the cloud.
As we saw in the first post of this series, the tech giants tend to know what they’re doing when it comes to AI. If you can leap your way over the governance hurdles and responsibly migrate PHI into the public cloud, you’ve gone a long way to solving your infrastructure issues. US healthcare providers probably have a big advantage here, as regulatory frameworks stateside tend to be a little more cloud friendly, and they fall under the same laws as the major cloud providers.
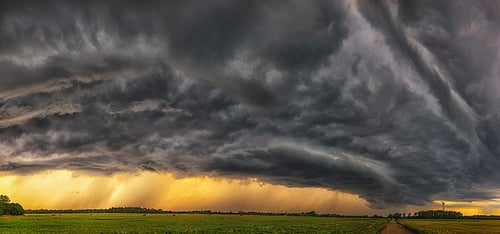
Of course, even once you’ve migrated to the cloud, you still need to address the governance issues around training AIs using PHI. But you’ll at least be part way there.
2. Create Trusted Research Environments.
TREs are places where researchers can access sensitive data and run various types of analysis. This can include training AI models. TREs are generally very secure facilities provisioned with specialist computing infrastructure you wouldn’t find in a clinical environment. PHI hosted within a TRE is either obtained with patient consent, de-identified, or even anonymised (in which case it is technically no longer PHI), so it’s easy to work with from a governance perspective. Consequently, there’s a big push towards TREs in the NHS right now.
A big appeal of NHS-hosted TREs is that it doesn’t require migrating PHI to the public cloud, which - as we’ve discussed - many centres are just not ready or willing to do yet. There are, however, some major downsides. Perhaps most notably for today’s discussion, the need to either consent patients or de-identify PHI bottlenecks the flow of data. De-identifying clinical notes is particularly challenging, and AI-based language models are particularly data-hungry, so it’s a double whammy for NLP applications like intelligent clinical search. That said, less stringent data protection regulation in the US has allowed researchers stateside to make good progress training clinical language models in recent years.
The other downside to TREs - particularly as part of a centralised, national model - is that they can become bogged down in bureaucratic and political challenges. For large academic medical centres, their clinical data is a huge asset. It allows them to produce high quality research outputs and attract funding. Handing that data over to centralised TREs where they don’t have a strong voice or significant vested interests can be a big ask, especially if the organisation administrating the TRE is perceived to be particularly bureaucratic or pursuing its own agenda.
3. Train and deploy AI in-house.
Now this is a really interesting approach. Probably the best example around is the London AI Centre's FLIP and AIDE programmes. The idea here is that a number of major London hospitals are installing specialist AI infrastructure within their own data centres - i.e. inside NHS firewalls. Some hospitals will have lighter-touch infrastructure that is only capable of “running inference”. Other, larger hospitals will install state-of-the-art AI servers that can train AIs as well as running inference. They’ll train models in partnership with leading London universities.
To ensure all Trusts with suitable AI training infrastructure can participate in training AI models without needing to share data with each other, they’ll use an approach known as federated learning. This is where an AI algorithm moves around from site to site, training on some of the data from each participating centre as it goes, but leaving the data exactly where it is. To ensure the PHI is de-identified from the AI’s perspective - which needs to be done for any “secondary use” of PHI under the terms of the NHS Data opt-out - they’ll use some clever tricks collectively known as “differential privacy”. Note that this only works if you’re leaving the data in situ; it doesn’t solve the governance problems of physically relocating data to the cloud or a TRE.
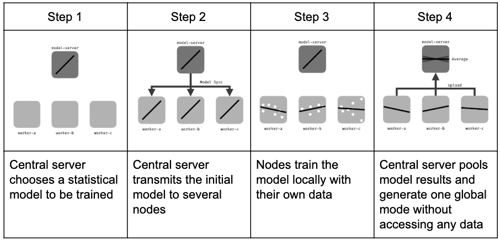
The brilliant thing about this programme is that it addresses all three of the major challenges we listed earlier. However, its biggest disadvantage is that it’s going to be very difficult to scale to less digitally mature parts of the NHS and other health systems.
At this point in time, it’s really not clear which of these three model will win out in the long run, if any. The one clear point of consensus is that we need to find a way to make PHI available to AI technology, and sooner rather than later. On which note, it's about time to recap what we've covered so far and wrap things up for the day.
What is the future of search technology in healthcare?
In part one of this series we talked about the impact that search technology has had on modern life. We saw how it is only going from strength to strength, with bleeding edge semantic search capabilities allowing for direction question-answering. We sketched out the kind of impact modern search could have on healthcare, if only we can find a way to bring advanced search capabilities to our non-internet-based data.
We explored how search technology works - both from a traditional, lexical search perspective and from a modern, AI-enabled semantic search perspective. We noted that next-generation internet search engines use AI to “read” web pages then index and rank them according to semantic content, not just the frequency of word occurrences. We concluded that, if we want to apply Google-type search capabilities more broadly at the point of care, we need to find a way to expose healthcare data to AI algorithms.
In this final post, we’ve talked about infrastructure and governance as two key barriers to connecting AI technology and PHI. We’ve looked at some of the models for addressing those challenges, each of which has its pros and cons.
So what’s next?

It’ll hardly surprise you to hear that the call to action from this series is to get started with the low hanging fruit. My job at Eolas is to bring advanced search capabilities and other AI-enabled technologies to bear on the non-patient medical information that we manage for our customers. If you want to hear a bit more about our platform and how it could improve your efficiency, we’d love to hear from you: hello@eolasmedical.com
In terms of bringing intelligent search to PHI, it's a case of watch-this-space. It'll be well worth keeping an eye on some of the groups we mentioned earlier in this post and who are continuing to trail blaze. For example, the UFHealth / NVIDIA team that already trained the world's largest clinical language model is rolling out an even bigger and better clinical language model in the near future. This could be key to overcoming the challenge of “domain shift”, which will be a big step towards more effective AI-enhanced clinical search. For some an update on the in-house approach to training and deploying AI models, you could check the series of webinars that the London AI Centre is running about delivering AI for the NHS. And, of course, you should definitely watch out for future blogs from us here at Eolas!
Well, that’s a wrap for this blog series. Please do get in touch if you’ve enjoyed this or if there are any particular topics you would like to cover in future.
Thanks for reading!